Let's Make Games
Cross-posted on the C&I substack
Everything you really want people to understand should be encoded as a video game. By this, I mean we make a game that's stimulating (and potentially even enjoyable), where understanding the core concept is on 'the critical path' to playing well.1
Thanks for reading! Subscribe for free to receive new posts and support my work.
Here's how I'm starting to think of it: take Bruno de Finetti's classic "Dutch Book" argument—probability theory is just that which you couldn’t bet against and win. Probability theory is on the critical path to any game of chance—how could it not be? But there are lots of other kinds of games, and we can make other concepts the necessary bottlenecks. To solve social games, you need social skills. To solve Chants of Sennaar you need to understand certain regularities in language use to decode the unknown languages you're immersed in.
Early education video games tried to do this, and while they managed to hook some kids, they were too unmixed: fun minigames and narrative sitting awkwardly next to frustratingly obvious "learning opportunities," but not integrated.
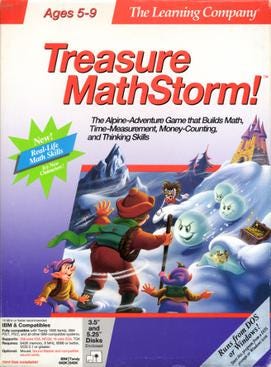
The education part was essentially a gate for the rest. We want everything mixed, deliciously cerebral peanut butter. Mmm…
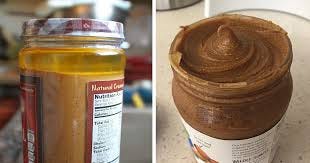
The point isn't just to incentivize people to learn, which is how I've generally seen this portrayed. The point is that when you play with something you understand it "in your heart" more than "in your head." You can write down the equations for kinetic energy (KE = ½mv²), but playing a racing game really makes you feel that v². We don’t want people who can fill out multiple choice questions correctly—we want people who use a concept naturally, intuitively.
Want people to understand social nuance? Make a game where you have to be the right amount of complimentary and firm to prevent an argument.
Want people to see why the prisoner's dilemma is hard to escape without precommitment? Make that a bottleneck to a multiplayer game.
Want people to understand why people develop a passion for trading and markets? Jane Street already did this.
I'm not sure that every concept can be made into a stimulating game, but I certainly think a lot more of them can be than most people think. Factorio, a game about building and automating factories, hardly sounds like a hit from that level of abstraction, but it is. Most things in life are about practicing and then performing, and quite a fecw aspects of that are fun.
Let's make games.
1 Thanks to Raul Castro Fernandez for the "critical path" terminology and always being more articulate than me.